Healthcare Appointment Scheduling with AI
- Home
- Healthcare Appointment Scheduling with AI
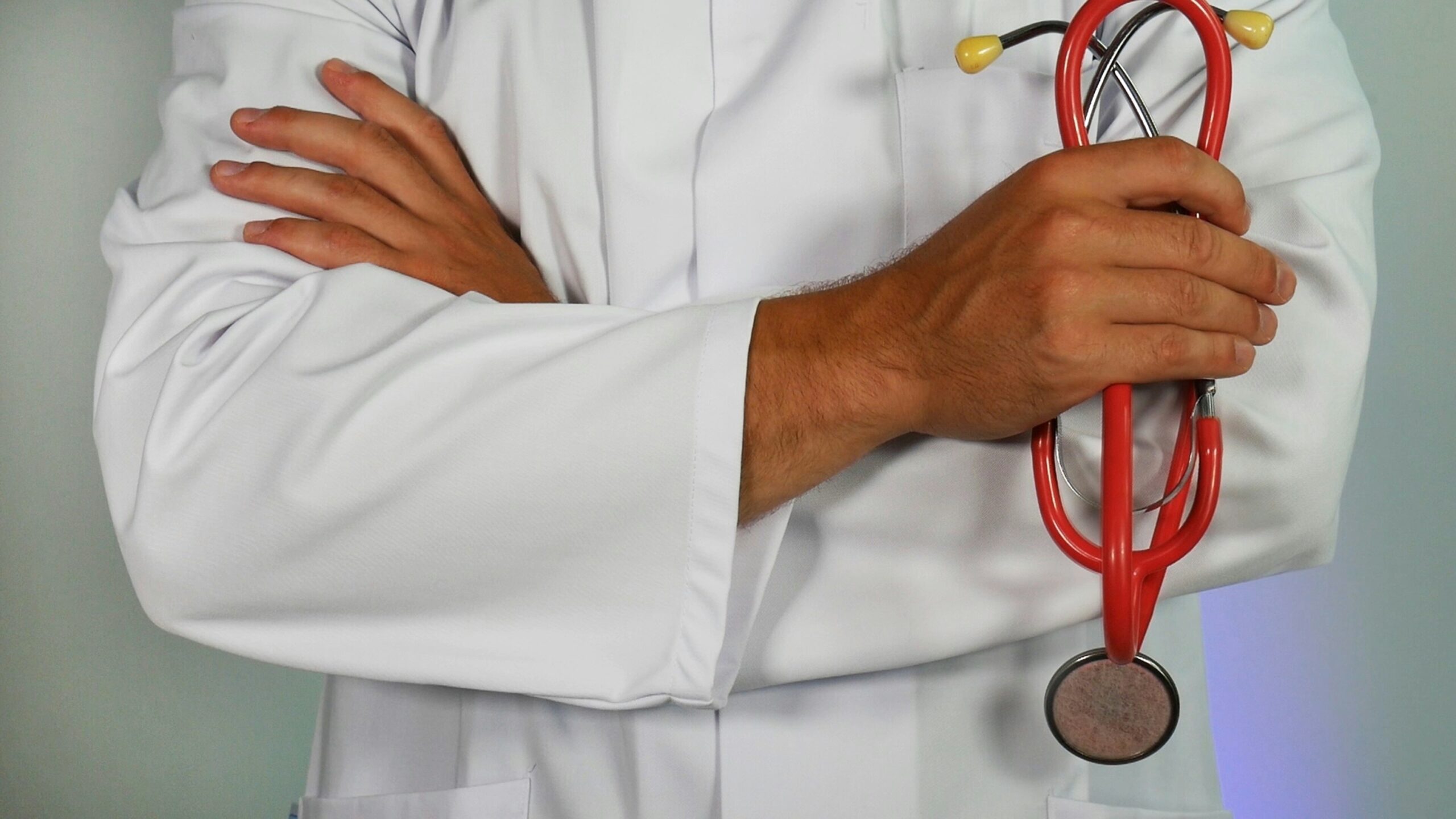
Healthcare Appointment Scheduling with AI
Introduction
Fusion AI Labs specializes in crewating cutting-edge, AI-powered solutions to transform industries. In 2024, Fusion AI Labs undertook a project to address a persistent issue in the healthcare industry: the inefficiency and complexity of scheduling appointments based on calendar availability. This case study highlights the research, design, and implementation phases of the solution, demonstrating how Fusion AI Labs streamlined healthcare appointment scheduling using AI-driven technologies.
Problem Statement
Healthcare providers often face significant challenges in managing appointment schedules. Patients struggle with:
- Limited transparency: Difficulty accessing real-time availability of healthcare professionals.
- Inefficiency: Time-consuming phone calls and manual interventions to secure slots.
- No-shows and cancellations: Lack of automated reminders and alternative slot management.
On the provider’s side, challenges included underutilization of time slots, administrative overheads, and errors in manual scheduling systems. These inefficiencies impacted both patient satisfaction and operational efficiency.
Research Phase
To design an effective solution, Fusion AI Labs conducted an in-depth study, including:
1. Market Analysis:
- Evaluated existing scheduling platforms and identified gaps such as limited customization and inadequate calendar integrations.
- Reviewed statistics showing 30% of patients missed appointments due to inefficient scheduling.
2. Stakeholder Interviews:
- Engaged healthcare administrators, doctors, and patients to understand pain points.
- Gathered insights into desirable features, including real-time updates, ease of use, and integration capabilities.
3. Technology Assessment:
- Assessed existing tools and APIs for calendar management (e.g., Google Calendar, Microsoft Outlook, healthcare EMRs).
- Identified potential AI algorithms for prediction and slot optimization.
4. Competitive Analysis:
- Benchmarked competitors’ systems, identifying lack of personalization and AI-driven automation as common shortcomings.
Solution Design
Fusion AI Labs’ solution incorporated the following key components:
1. Dynamic Calendar Integration:
- Leveraged APIs to integrate seamlessly with providers’ calendars (e.g., Google Calendar, Office 365).
- Supported multi-provider and multi-location scheduling.
2. AI-Powered Slot Optimization:
- Implemented machine learning algorithms to predict high-demand periods and optimize slot allocation.
- Introduced features to recommend slots based on patients’ preferences and historical behavior.
3. Integration and Automation The solution was integrated into existing EHR systems, offering:
- Designed an intuitive web and mobile app for patients to view real-time availability and book appointments effortlessly.
- Included support for rescheduling, cancellations, and waitlist management.
4. Automated Notifications and Reminders:
- Developed an AI chatbot to send reminders, handle queries, and confirm appointments.
- Added email and SMS notifications to reduce no-shows.
5. Data-Driven Insights:
- Provided analytics dashboards for healthcare providers to track appointment trends, no-show rates, and resource utilization.
- Admin portal for doctors and staff.
- Patient-facing web application for scheduling and managing appointments.
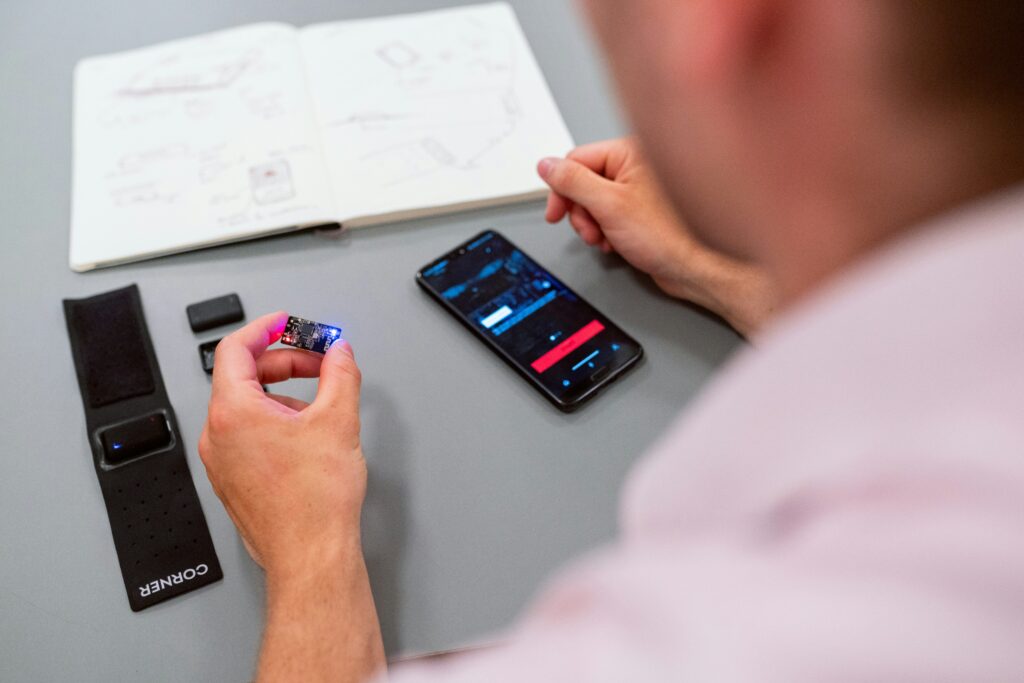
Implementation
Phase 1: Prototyping and Testing Fusion AI Labs developed a prototype and conducted usability tests with a pilot group of healthcare providers and patients. Feedback included:
- Enhancing mobile app responsiveness.
- Streamlining the onboarding process for providers.
Phase 2: Deployment After refining the solution, Fusion AI Labs deployed it for multiple healthcare providers, ensuring:
- Secure integration with existing EMR systems.
- Compliance with healthcare data privacy regulations (e.g., HIPAA).
Phase 3: Optimization Post-deployment, the solution’s machine learning models were fine-tuned based on real-world data to enhance prediction accuracy and slot allocation.
Results
1. Patient Satisfaction:
- 85% of patients reported an improved scheduling experience.
- 25% reduction in appointment no-shows.
2. Operational Efficiency:
- Administrative workload reduced by 40%, allowing staff to focus on patient care.
- Slot utilization improved by 25%.
3. Scalability:
- The solution was scalable, accommodating healthcare practices of varying sizes.
- Supported over 10,000 appointments within the first six months.
Conclusion
Fusion AI Labs successfully addressed the healthcare industry’s scheduling challenges by combining AI-driven automation, user-centric design, and seamless integration. The solution not only improved patient satisfaction but also enhanced operational efficiency for providers. This project exemplifies Fusion AI Labs’ commitment to delivering transformative AI solutions that address real-world problems.
Future Enhancements
To ensure continuous improvement, Fusion AI Labs plans to:
- Incorporate voice-activated scheduling through virtual assistants (e.g., Alexa, Google Assistant).
- Use predictive analytics to forecast long-term scheduling trends.
- Expand the solution to other industries such as dental care, physiotherapy, and telemedicine.